Signals and sensations
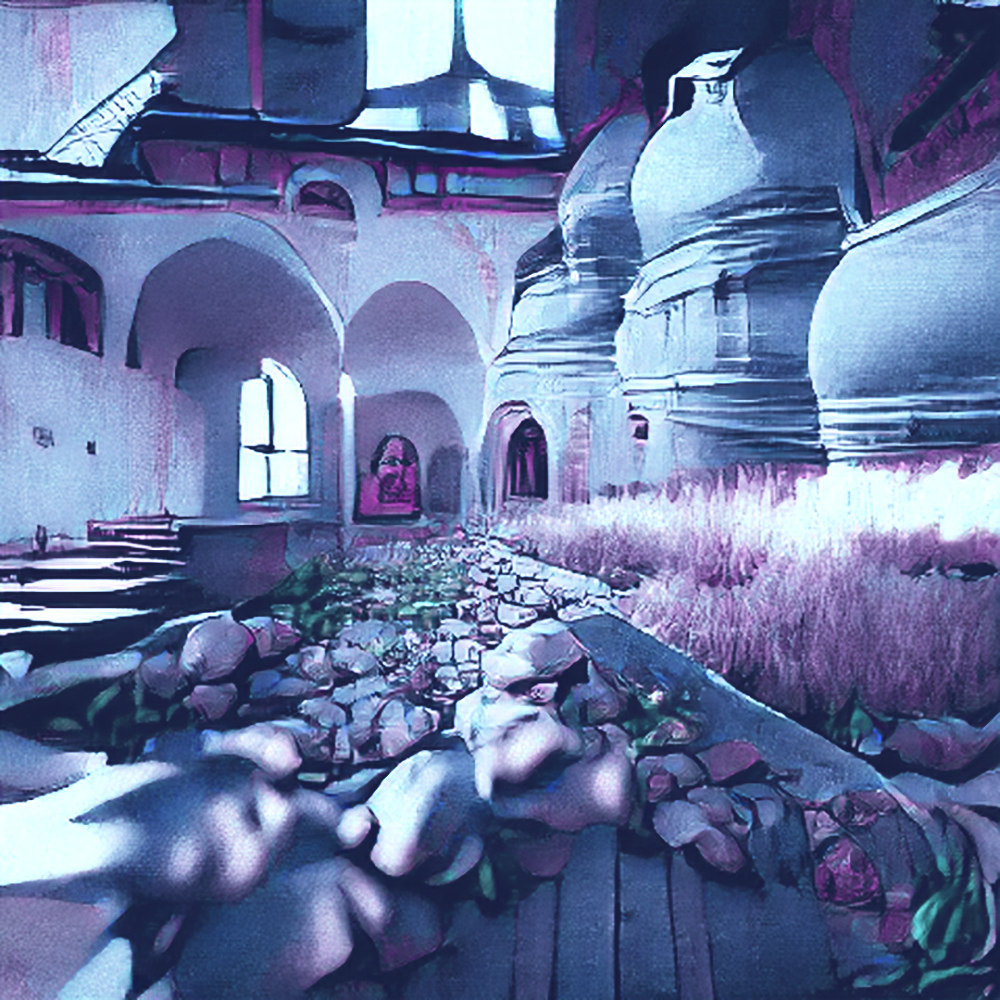
In order to perceive stimuli from the environment, people have sensors in the form of sensory organs. Information received through these is often incorrectly referred to as perception. Different disciplines such as psychology, physiology, biology and philosophy define the term perception differently. In both psychology and physiology, perception refers to those sensory stimuli that are processed cognitively. Perception therefore describes the sum of reception, selection, processing and interpretation of sensory impressions. Incoming information or stimuli are converted into a signal by the sensory cells, received by nerve cells and transmitted as an electrical impulse via nerve fibers to the human data center, the brain. The conversion of a stimulus into a potential is called transduction. What stimulus is actually received by our sensory organs varies. Whether a stimulus triggers a sensory sensation depends on whether it exceeds a difference threshold, i.e. the smallest perceptible difference in the physical intensity of two stimulus presentations. Even during transmission, signals from the sensory organs in the nervous system can be compared and compared, combined, filtered or contrasted. The information that is transmitted to our brain is often referred to as sensation. Thus, objectively identical stimuli from the external world can lead to subjectively different sensations.1
perception
However, sensations are not perceived unfiltered. The incoming information must first be processed in order to make it usable. This can lead to strong deviations in the perceived sensations.
An example of this is the perception of colors. In 2015, the colors of a dress were discussed under the hashtag Dressgate. Twitter users perceived the colors in a picture of a dress differently. The reason for this is that our brain evaluates the color information depending on the surrounding daylight. This color correction causes the colors to be perceived differently. Colors are essential for how we perceive our surroundings and interpret information in them. At the same time, however, we know that our brain assembles this information for us from electromagnetic radiation in different wavelengths. Despite this knowledge, colors are no less real or meaningful to us.
There is an explanation for the different perception of the colors of the dress. But how do we know that the item is a dress? We have already seen clothes and the object in said picture is similar to what we already know. What would happen if the dress was described in a text? Hermann Helmholtz's theory of perception psychology, developed in 1866, states that we use our experiences to unconsciously draw conclusions about what we perceive. This enables us to perceive quickly and effectively. However, this can also lead to us misinterpreting new situations. According to Helmholtz, only signs enter our perception that never represent an image of what we perceive:
“Insofar as the quality of our sensation gives us information about the peculiarity of the external influence by which it is excited, it can be considered a sign of it, but not as an image. Because one demands from a picture some kind of similarity with the object depicted, […] from a drawing, equality of the perspective projection in the visual field […] But a sign does not need to have any kind of similarity to the thing whose sign it is. The relationship between the two is limited to the fact that the same object, acting under the same circumstances, produces the same sign.”2
Hermann von Helmholtz described a signal stream over 140 years ago that flows not only from the sensory impressions of the outside world to the brain, but in the opposite direction. According to Anil K. Seth, who studies the biological basis of perception at the University of Sussex, our perception relies on both of these signal streams:
“If we think of perception as a direct window into an external reality, it seems logical that information flows from the sensory organs to the brain, i.e. from the bottom up. Signals directed in the opposite direction could only contribute connections or refinements to what is perceived - nothing more. In such a view, it seems as if the world reveals itself to us directly through our senses.
The scenario of the prediction machine is completely different: Here the main part of the perceptual activity is carried out by signals directed from top down (top down), which provide predictions about the perception. The stream of sensory impressions directed to the brain only serves to refine these predictions and to connect them appropriately with their real causes. Our perception therefore relies at least as much on a flow of information directed towards the periphery as the other way around, if not more so. So it is not a passive reception of an external, objective reality, but an active construction process - a controlled hallucination.3
Our perception involves a wide variety of processes for processing, organizing and interpreting sensory information that take place in our nervous system and our brain. A wide variety of brain regions are involved in this. Since our brain was identified as the seat of our cognitive performance, comparisons have been made between the human brain and the most complex technical devices currently available. Today we compare the brain with the most powerful computers we know and, conversely, attempts are made to recreate computers using human neural networks. However, in contrast to some technical information systems, the goal of our brain is not to record as much data as possible. Our brain activity even drastically minimizes the amount of incoming data - without us being aware of it - to a level that we can process. Incoming information is reduced to a ten-millionth of its quantity. When output through language, facial expressions or general motor skills, information is enriched with the brain's own information through association processes. This reduction process represents a kind of bottleneck through which the flow of information has to pass.4 Even if we see the same object, we will never perceive it the same way or describe it the same way.
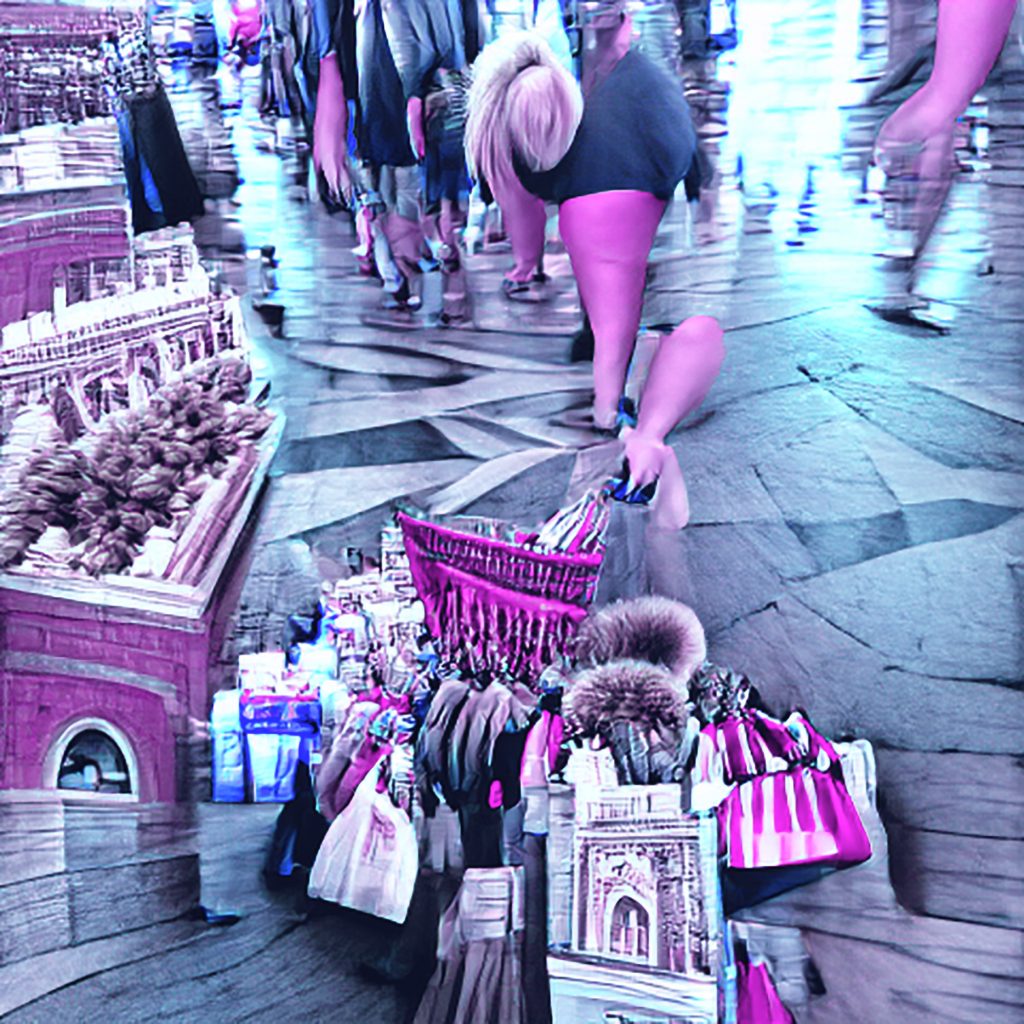
Your counterpart didn't understand what you meant.
As you sit down at your desk, the lump in your throat moves towards your abdomen and gets stuck there.
You're looking at a meme on your phone that features Spock from Star Trek. Memes are a universal form of communication, but one that is conditioned by the ability to refer. You smile. You are amused because you know that Vulcans are a fictional species who have managed to suppress their emotions and think and act purely based on logic. Vulcans are telepaths and can transmit their thoughts directly and unadulterated to other people. Optimal. Talking to each other is more difficult. Even if the other person is ready to receive a message, the sender can never determine whether it will be evaluated as desired. The more you observe this, the more attractive communication with machines appears. Your computer only knows zeros and ones. No matter what you enter, it will be evaluated exactly according to the input, without the addition of emotion and endless referencing to previously acquired knowledge. You open your laptop.
communication
Knowledge about information processing, its reduction and interpretation, and the consideration of individual perception as a controlled hallucination allow us to better understand why interpersonal communication often fails. We cannot fall back on a shared understanding of objective reality. And even more fatal: our brain uses certain rules of thumb and shortcuts with which it tries to make useful decisions and simplify complex problems in a limited time based on limited knowledge. The art of arriving at probable statements or workable solutions in a short time and based on incomplete information is called heuristics.5 But our brain, as a prediction machine and friend of simple heuristics, not only constantly creates new hypotheses about sensory impressions, but also constantly optimizes their prediction. It is able to use a feedback loop of what was predicted and what actually happened to determine a prediction error, which it can apply to the next forecast. Although the brain is designed to keep the deviation between the predicted and the actual sensory impression as small as possible, it also optimizes assumptions based on new data.3
These peculiarities of the brain result in various obstacles to interpersonal communication, but also the ability to question one's own perception based on new data. In English, communication obstacles are often referred to as cognitive biases. In sociology, this refers to cognitive distortion, which is a collective term for systematic, unconscious and incorrect processes in human information processing - our perception, memory, thinking and judgment.6 They are based on the predictions described above and represent so-called cognitive heuristics. For example, people tend to perceive arguments that speak for their own opinion as stronger than arguments that speak against their own opinion. This is known as confirmation bias. Additionally, we tend to consume information that is consistent with our existing worldview. Social psychologist Ziva Kunda described this phenomenon as motivated reasoning - a mutual influence of motivation and cognition that can lead us to hold on to false assumptions despite evidence to the contrary.7
The term filter bubble, coined by internet activist Eli Pariser in 2010, describes a state of intellectual isolation. Filter bubbles are created by selecting received information based on the evaluation of search queries, location or user behavior through algorithmic predictions made on websites.8 Our natural predisposition to want to maintain existing world views, opinions and ways of looking at things and the systematic pre-selection of incoming information favors one-sided perceptions in this context. From this point of view it becomes increasingly difficult to understand other points of view and views.
Prejudices can also be better understood with knowledge of the processes in our perception, because their maintenance is often subject to bias. In social psychology, an attribution error, also known as correspondence bias, is “systematically overestimating the influence of dispositional factors, such as personality traits, attitudes and opinions, on the behavior of others and underestimating external factors (situational influences)”.9 We try to explain a person's behavior based on their membership of a certain group, be it a certain social group, a political party or simply the person's origins. This type of subconscious and automatic thinking process is called a judgment heuristic.
Our thinking and perception processes are responsible for all sorts of side effects. They cause misunderstandings, insults, conflicts, or even violence. However, they also allow us to reflect on our perception. This empowers us to improve our communication, question our perception, or even conduct perception experiments.
Your news feed knows what might interest you. During your lunch break, you prefer easy-to-digest bits of information. Tech News.
“Recognition of bias in AI continues to grow”, “Facebook Apologizes After AI Puts 'Primates' Label on Video of Black Men”, “Explained: Why Artificial Intelligence's religious biases are worrying”… Not the desired results.
You don't have to fully understand how artificial intelligence works. It is enough to be certain that it is just mathematics with certain extras.
The extras seem to inject all human bias and misinformation into your digital sanctuary. The abdominal dumpling that was thought to be digested becomes a yeast dumpling and bloats up. You escape to the roof terrace of the university. It's cool and windy there. If learning leads to referencing and that leads to prejudices and misunderstandings, it might be wise to stop learning.
Artificial intelligence
»“I was in a room with a scientist who wanted to demonstrate AI to me. He pulled out a box and said, “This is your AI.” I was quite amazed. "Is this a joke?" I asked him. “No,” he replied, “the AI will be what you think.” So I said, “It has to be a dog.” And at that moment the box changed and turned into a dog. It was adorable and looked like my own dog, who had recently died, so it seemed perfect to me. As I bent down to pet it, the scientist took it out of the room and put it back on the table. “The dog is not an AI,” he said seriously. "What?" I asked incredulously as he grinned knowingly at me. “You told me the AI would be what I thought it was!”
“There is no such thing as artificial intelligence,” he explained.
»Artificial intelligence is the intelligence demonstrated by machines, in contrast to the natural intelligence of humans and other animals. Artificial intelligence is a field of computer science and engineering that aims to create computers and computer programs capable of making decisions and completing tasks similar to humans.
AI can be described as the ability of a machine or software to imitate intelligent human behavior, such as problem solving, pattern recognition, speech recognition, language translation, decision making and learning. Deep learning is a subset of artificial intelligence that includes algorithms that learn tasks by analyzing large amounts of data.
In the 1950s, the first generation of AI scientists focused on an approach called “symbolic” AI, in which machines are programmed with knowledge about the world in the form of symbols or representations. For example, a word processor can be automated to change words to improve spelling or grammar by telling it what each word is. However, this symbolic approach to programming has its limitations. Although it can automate certain tasks that were once limited to human work, it still has a limited understanding of how humans think and experience emotions.
This changed in 1995, when a team at Carnegie Mellon University developed a new machine that could play chess better than any human had ever done before. This machine differed from previous computers because it was not programmed with game rules, but rather learned by playing against itself. The machine learned from its experiences over time - just like humans do - which dramatically improved the way it played chess. Artificial intelligence, also known as AI or AI, can be explained conclusively and simply. It's about so much more than automating everyday tasks. It's about creating simulated environments for technical, medical, cognitive and military purposes. It’s about understanding the world through calculations.«
The last two texts have in common that both were created by an AI tool for automated text generation. To do this, the user enters keywords or sentences - a so-called prompt - which the text generator expands or on the basis of which it creates a completely new text. For the first text, several prompts were used, the results or excerpts of which were rearranged to create a coherent text. The second text was generated by the AI tool Rytr exactly as shown.
The AI writing assistant is able to formulate both factual descriptions and fantastic stories. Using large amounts of data, it learns how likely two words are to follow each other. So he doesn't use any prefabricated text modules, but rather selects each word based on a probability distribution. The check using plagiarism detection software showed that no identical sentences or text modules could be found for either text by a search engine.
When it comes to content, the generated text is surprisingly precise, but not entirely correct.
The term artificial intelligence was actually coined in 1955 by the American computer scientist John McCarthy, the inventor of the LISP programming language, who used it in a funding application for a research project. He deliberately chose the term to distinguish his work from the research field of cybernetics.10
The chess computer mentioned also exists. In 1949, Arthur Samuel began his research in the areas of AI and machine learning. In 1952 he developed a chess-playing program for the IBM 701 mainframe computer. The program selected its moves based on a predictive search in a so-called search tree and thus achieved good playing skills. Today this is called a heuristic search method.11
However, the chess computer called the AI Writing Assistant, which was developed by Feng-hsiung Hsu at Carnegie Mellon University, dates back to the 1980s. In 1989, the computer called Deep Thought won the World Computer Chess Championship. However, Deep Thought didn't always beat his human opponents. It wasn't until 1996 that his further development, a new chess computer called Deep Blue, managed to beat the then world chess champion Garry Kasparov.
The further development that the AI writing assistant represents in the area of game processing is based on the development of a symbolic AI (top-down process) towards a neural AI. Bottom-up processes are aimed for. So you are no longer limited to “manually programmed” heuristics, but rather aim for heuristics “learned” by the AI itself. In addition to large amounts of data, this also requires high computing power. Technological progress is opening up ever better learning opportunities for AI.
In addition to game processing, AI is also used for image processing, language processing and many other areas. The resulting output always depends on the information fed in for learning, human-made information. Malfunctions, biases and undesirable behavior of AI are regularly reported in various media. These often imply that humanity has entered a collective state of the sorcerer's apprentice, who, having become powerless over his creation, has to watch it work. As with any other tool, this technology is only as good as its users. Those algorithms that relegate us to a filter bubble could also detect disinformation or cognitive, social or algorithmic echo chambers. How these technologies are used also reflects the values of the corporations that use them for their purposes.
Artificial intelligence can also be used in architecture. Construction projects, planning processes and urban structures are becoming increasingly complex and the amounts of data to be processed are becoming larger. It seems almost inevitable to use new technologies in this field too. In addition to options for efficient administration and communication, there are AI-supported tools that can be used for the design process or provide answers to recurring, frequently occurring planning tasks. A distinction can be made between parametric, generative and AI-supported design.
An example of the latter are so-called Self-Organizing Floor Plans (SOFP), which are able to adapt and optimize floor plans to a wide variety of specified parameters.12
In parametric design, geometric specifications are programmed. Code can be written yourself or created using a visual programming language (VPL). With visual programming languages, users can put together code from graphical building blocks, so-called nodes, without having to write code themselves. An example of this is the visual programming language Grasshopper 3D. This procedure is analogous to classic programming based on rules. However, similar to the example of the first chess computers, all parameters must be specified manually. As soon as more or more complex parameters are required and the design has to react to rapidly changing conditions in the outside world, difficulties can arise.
Generative design uses genetic optimization algorithms - algorithms inspired by the evolution of natural creatures that evolve (optimize) artificial neural networks - which are applied to parametric designs to select input parameters based on a target. This makes it possible to generate an output based on much more complex and extensive input.13
One criticism of parametric design is that the limited input parameters often only serve to achieve a certain aesthetic. As part of his teaching at the Linz University of Art, the Berlin architect Tobias Hönig was critical of the use of parametric design, which, in his opinion, was used exclusively to achieve extravagant shapes using random parameters without a comprehensible origin or information content.
A project that can be distinguished from this is R&Sie(n)'s “I've heard about it…”. The Paris architecture firm creates a model that does not aim to represent an actual city, but rather generates a so-called metamodel. A habitable organism - an adaptive landscape in a constant state of development - is created using information from its inhabitantscreated inside. The information used for this is obtained through nanoreceptors that respond to chemical emissions from the residentsreact internally that are triggered, for example, by stress or fear. This data is fed into the VIAB engine (of viability and variability). This is a robot developed by the Robotics Research Lab at the University of Southern California that secretes fiber cement and produces the generated structure. The VIAB machine is part of the landscape in which it moves and which it creates.14 Felix Guattari, one of the co-founders of the studio R&Sie(n), describes the metamodel as “the mathematical prehension of an urban system that is simply relatable to the order of data structures, to the abstract dimension of numbers and their autonomous connections and disconnections, and which is neither driven by a pre-established logic nor by an external set of concrete influences. I've heard about it…, therefore, is the metamodel of a city whose data architectures do not model an existing urban space but simply construct its order. The project, in other words, has no references to predetermined ideas or to the concreteness of reality, but simply describes the conceptual prehension of an 'architecture of abstraction'”.15
This type of approach could be used to visually represent connections and processes in real urban spaces and to make complex system processes more tangible.
Generative design is also used in traffic and mobility planning. Genetic algorithms are able to evaluate traffic data and create behavioral models to analyze different traffic flows. Such behavioral models can analyze the driving behavior and route selection of individual road users and predict the impact of interventions in the existing system. This can increase the resilience of transport networks and facilitate urgent decisions about changing transport networks, for example in the event of natural disasters.16
Research into artificial intelligence is constantly offering a lot of new things and is constantly expanding. Even if many areas of use still seem futuristic and far away, there are opportunities for everyone to experiment with AIn who has a computer or smartphone with internet access. AI text generators, like the program that generated the introductory texts of this chapter, are available as freeware or trial versions. It is also possible to generate images using services on the PC or smartphone. Various apps turn entered text into AI-generated images. Google Colaboratory also enables this with code provided by the authorsis made available inside so-called notebooks, and images can be created from text specifications without much prior knowledge.
You walk through the city. The city sends you a constant fire of stimuli. The city sends visual stimuli, audible stimuli, tactile stimuli. It communicates with you through its buildings, its inhabitants, its topography, its squares, streets and alleys. Most of the time you understand the city. You know the behavioral norms and processes embedded in them. Some scenes play out the same way over and over again. The tram pulls into the stop. The doors open and you get in. Warm draft. You choose a window seat and stare into space somewhere between the glass window and the outside world. Your gaze is interrupted by a sudden irritation. A small short circuit is triggered in your thalamus and sizzles there briefly before it dies down. And just like that you drove past the scene. However, you can still access the image like a photo. An involuntary screenshot has burned into your hard drive memory. What was that thing at the intersection? That must have always been there. It didn't look new. A mixture of curiosity and uncertainty is spreading. Your smartphone knows all the streets in the city and it even has pictures of them. Street view. There it is! This thing seems to break through its surroundings. His differentness makes you suspicious. Your synapses, formed in the last five minutes, launch a DDoS attack on all established members of your species. It can never work like that. And if it works like that, why does it have to look the way it does? Who created it? And when and with what right? “Next stop, Linz main square, art university…”. Get up, get out. Cool air wafts through the doors and hits your face before you've even fully stepped off the tram into the new environment of the main square. Is it still charging, or does it always look like this?
New realities
In addition to the perceived external world, content from media such as films, photographs, books or video games are also impressions that we use to construct our reality. Although we have not experienced their content ourselves or know that they are purely fictional in nature, they serve as a reference when we encounter something new. By consuming these media, by reading, playing, listening and watching, we expand our reality and learn. The English word immersion describes immersion in a virtual environment. The term is best known in connection with virtual reality, but you can also immerse yourself in films or books. What VR has going for it is the ability to create a 360° comprehensive virtual environment.
In recent years, our physical reality has begun to increasingly mix with digital content. Mixed reality and augmented reality do not create a completely new environment, but rather integrate digital objects into the natural environment. Through glasses, headsets and even smartphones, digital content can be projected into our physical environment and is even interactive.
An experiment at the University of Sussex shows that people can perceive a virtual space as completely real. Panoramic images of a laboratory were taken for the experiment. Test subjects were equipped with a VR headset with a built-in front camera. While the test subjects looked around the laboratory through the headset, the previously recorded panoramic image was shown on the headset instead of the image from the front camera. Most of the test participants continued to perceive what they saw as real. It becomes clear that, under certain circumstances, people can be deceived into not being able to distinguish VR content from their actual surroundings.3
A similar experiment, also carried out by the University of Sussex, involves the creation of a hallucination machine. For this purpose, external photographs were taken on the university premises and analyzed using an algorithm based on the DeepDream program, which is used to recognize and classify images. For example, the program recognized different dog breeds. The algorithm's working method was then changed so that the output updates the input, i.e. it runs backwards, so to speak. This resulted in objects being projected onto the footage that the algorithm believed were there. If this process is carried out several times, more and more distorted images appear in places predicted by the algorithm. This resulted in a video in which dogs appeared in various places because the algorithm predicted them at many more points than where the dogs were actually present. The video footage created during this experiment appears surreal and psychedelic, resembling a dream or hallucination.17
Looking at this type of imagery triggers unfamiliar feelings in us. In English this is described as eery or uncanny (sinister, mysterious). The term Uncanny Valley describes an effect that artificially generated images, video games, films or even robots have on us. One could assume that acceptance of avatars in video games, for example, increases the more realistically they are portrayed. However, this acceptance does not increase monotonically with anthropomorphism, i.e. the similarity to humans, but decreases sharply at a certain point. We perceive highly abstract and artificial figures as more likeable and are more likely to accept them than figures who are located exactly in this valley between abstraction and realism, the uncanny valley.
Another phenomenon that triggers similar sensations and can also be found in video games, or is consciously taken up by them, are liminal spaces. The term liminality, coined by the ethnologist Victor Turner, originally describes a threshold state of individuals or groups who have ritually separated themselves from dominant social order systems and are in a kind of threshold phase before being reintegrated.18 The term liminal space, derived from this, describes border spaces that form a transition or passage between two places or states. These are often empty or abandoned. Typical examples are abandoned shopping centers, empty open-plan offices or school rooms during school-free periods. However, the aesthetics of liminal spaces are broader and can describe not only liminal spaces, but also the appeal of images based on nostalgic emotions. Liminal spaces are strongly linked to the cultural memory of generations Y and Z. The special aesthetic is therefore also influenced by elements from the aesthetic movement of vaporwave, which uses elements and colors from trends from the 80s to the early 2000s and often deliberately exaggerates them. The word glitch can also describe a special type of image aesthetic that is based on image errors in films or video games and is also used to underline the aesthetics of liminal spaces. Liminal spaces are mostly depicted in photographs, but it is also possible to generate them artificially. Renderings of Liminal Spaces can be created in 3D graphics suites such as Blender. The resulting images are often given additional blur or a gloomy lighting situation in order to achieve the effect of a liminal space.19
Usercollect and publish images of Liminal Spaces on various websites. Also AI-generated images from users' text promptsarise within, are shared. The AI image generators use algorithms for unsupervised learning - learning without a previously known target value and without external reward - for example VQGAN (Vector Quantized Generative Adversarial Network) in combination with CLIP (Contrastive Language–Image Pre-training).
GANs (Generative Adversarial Networks) are systems in which two neural networks react to each other. One of the networks – the generator – creates images or data. A second network – the discriminator – evaluates the results. Thus, the system reacts to itself to improve its results. CLIP is an attendant neural network that finds images based on a text description that is fed into the Generative Adversarial Network.20 The video and image material generated in this way ranges from grotesque, difficult-to-interpret outputs to high-resolution portraits of people who never really existed.
Where the glitch lives
The glitch is a short-term false statement in a logical system. It is a manifestation of an illogical or flawed condition, system or programming that underlies it. In this way, the glitch can be clearly distinguished from a so-called bug, i.e. a programming error. Alex Pieschel, a writer for Arcade Review, describes the difference as follows: “'Bug' is often cast as the weightier and more blameworthy pejorative, while 'glitch' suggests something more mysterious and unknowable inflicted by surprise inputs or stuff outside the realm of code."21
You sit down on a park bench and close your eyes. Your world is a dull black punctuated by moving, geometric shapes in neon colors. You slowly open your eyes. Your reality becomes colorful and loud. Information bombards you at 109 bit/s. You successfully reduce it to 102 bit/s. Breathe in. You enrich the information to 107 bit/s through association processes. Exhale. Your right brain dances the tango.
In our cities, a glitch can be an artifact left in a new environment without a purpose. The glitch can also be a new object. It can be a mood, a traffic route or a use. The Glitch is the protagonist of a tragicomedy who needs an audience. The glitch is the brief, strange moment of irritation when we fail to clearly reference something. The glitch can be a thing, a building, a room, a district, a system or a complete situation. What is perceived as a glitch varies and depends on personal experience and level of knowledge, as well as on the individual concept of normality and reality.
If you transfer the phenomenon of the urban glitch and its strange effect to our perception, this means that similar sensations are triggered by objectively completely different stimuli. There is no other known sign of the glitch that can enter our perception instead of the image of our sensation. The task now is to find a known sign and tolerate a deviation between sensation and perception, or create a new sign. Both bind our sensations to laws and an existing system of perception order. The glitch as a sign itself enables an unbiased classification of these new impressions. The designation as a glitch denotes a perceived irregularity and warrants a closer look and investigation. The original use of the word glitch in technical disciplines opens up a new vocabulary that serves as an analogy to describe the urban glitch. Investigation and planning methods from other disciplines, such as software development, can also be used to investigate a glitch. This makes it possible to deal with complex or even depressing topics from a new perspective and to communicate the insights gained in an unbiased manner.
The glitch came from technology. You saw it and packed it up. When you live in a hallucination, you want to hallucinate the way you want. In your bag with the glitch you stuff new words and a few tools, two flowcharts and a shoebox of error messages. Now you're pretty powerful. With the things in your bag, you can show me a piece of your hallucination. You can also cut some of it off and distribute it.
You go to the Franz-Josef-Warte, scatter the box of error messages all over the city and scream: “Artificial intelligence doesn’t exist!” Then you laugh out loud.
2 Helmholtz, Hermann von. The facts in perception. Speech. Berlin: sn, August 3, 1878.
3 Seth, Anil K. Spectrum. [Online] February 1, 2020. [Quote from: December 23, 2021.] https://www.spektrum.de/news/unsere-inneren-universen/1696550.
4 Vester, Frederic. The art of thinking in a networked way. Munich: dtv, 2011.
5 Simple heuristics that make us smart. Gigerenzer, G. and Todd, PM New York: Oxford University Press, 1999.
6 Weber, Silvana and Knorr, Elena. The psychology of post-factual: About fake news, “lying press”, clickbait & Co. [ed.] Markus Appel. Berlin: Springer Berlin Heidelberg, 2020. P. 104.
7 The case for motivated reasoning. Kunda, Ziva. 1990, Psychological Bulletin, pp. 480-498.
8 Bias in algorithmic filtering and personalization. Bozdag, engineer. 2013, Ethics and Information Technology.
9 Aronson, E., Wilson, TD and Akert, RM Social Psychology. sl: Pearson Education Germany, 2008. P. 108.
10 Kaplan, Jerry. Artificial Intelligence: What Everyone Needs to Know. Oxford: Oxford University Press, 2016.
11 Sutton, Richard S. and Barto, Andrew G. Reinforcement Learning: An Introduction. sl: A Bradford Book, 2018.
12 Carta, Silvio. Self-Organizing Floor Plans. Harvard Data Science Review. July 23, 2021.
13 Architecture. Parametric Design, Generative Design and AI-Aided Design. . [On-line]
14 Canadian Center for Architecture. [Online] [Citation from: January 7, 2022.] https://www.cca.qc.ca/en/archives/464863/rsien-project-records/500303/ive-heard-about-and-hypnosis-chamber .
15 Parisi, Luciana and Portanova, Stamatia. Soft thought (in architecture and choreography). Computational Culture. November 2011.
16 Osogami, Takayuki, et al. Toward simulating entire cities with behavioral models of traffic. IBM Journal of Research and Development. September 2013.
17 Suzuki, Keisuke, et al. A Deep-Dream Virtual Reality Platform for Studying Altered Perceptual Phenomenology. Scientific Reports. November 22, 2017.
18 Lessa, William A. and Vogt, Evon Z. Reader in Comparative Religion. sl: Harper & Row, 1979. P. 234.
19 Aesthetics Wiki. [Online] [Quote from: January 3, 2022.] https://aesthetics.fandom.com/wiki/Glitch#Visual.
20 Burgess, Phillip. Adafruit. Generating AI “Art” with VQGAN+CLIP. [Online] July 21, 2021. [Quoted: November 18, 2021.] https://learn.adafruit.com/generating-ai-art-with-vqgan-clip.
21 Pieschel, Alex. Glitches: A Kind of History. Arcade Review. December 8, 2014